Lies, Damn Lies, and your Evidence Base
People can come up with statistics to prove anything, Kent. 40% of all people know that
Statistics are confusing. Any science that spawns phrase like "the presence of heteroskedasticty indicates that the disturbances are non-spherical" is bound to be mis-interpreted at some stage. Of course the same might be said of many technical disciplines but the fact that I don't understand the ins-and-outs of neuro-science presents me with little difficulty in my daily life. Statistics on the other hand, are everywhere! The following tips are aimed as the non-statisticians who's work requires them to interpret statistics.
I'm particularly thinking of policy makers who are faced with the task of gathering an evidence base to guide their strategies and actions. These warnings encourage a healthy degree of scepticism and give you a better idea of what questions to ask of your research. Before we begin, it's worth defining the word statistic. When we're describing something quantitatively we refer to parameters about the population; for example: "there are 10 cars on my street". When we don't know about the whole population (e.g. my neighbourhood), we have to look at a sample (e.g. my street). We can estimate the population parameter from the sample (e.g. there are 10 cars on my street, and 10 streets in my neighbourhood, therefore I estimate that there are 100 cars in my neighbourhood). The sample statistic (cars in street) used to estimate the population parameter (cars in neighbourhood).
- Statistics never prove anything! Let's be up-front about this. Statistics are not the truth but they can tell you about lies. Statistical inference is a process of elimination. I don't prove my theory, I just disprove everything else! Or as Sherlock Holmes would have it: "when you have eliminated the impossible, whatever remains, however improbable, must be the truth". In reality, it's impractical to eliminate all impossibilities. Our first health warning then, is not to take anything as given: question everything!
- What's the point in statistics? Because statistics are estimates that draw on a limited view of reality there is a risk that they'll give the wrong answer. This is known as sampling error. When you see a statistic presented you're typically looking at what's called a point estimate. This is the most probable value that we think the underlying population parameter will take - our best guess. Good statisticians provide confidence intervals along with their point estimates. These intervals give the range of values we expect the parameter could actually take. In order to really understand a statistic you ought to know range of possibilities. Technically the full range is infinite, but statisticians tend to allow some margin of error - such as a 5% tolerance for inaccuracy - giving them interval that contains the actual value 95% of the time.
- Expect the unexpected! A corollary of the second point is that statistics will be wrong 5% of the time. If your evidence base quotes 20 statistics then you can expect one of them to be wrong. This further illustrates the importance of the first point. The question is how rare is rare? Statisticians call the tolerance for inaccuracy (e.g. 5%) a type one error (excessive trust) - it is the likelihood that they'll erroneously reject the truth. The alternative is to ask "how much inaccuracy must I tolerate to accept this statistic?" which leads to the second type of error: failing to reject a falsehood (excessive scepticism). Health warning: You can't expect to be right all the time, but it helps to know how often, on balance, you'll be wrong.
- Bigger populations do not always need bigger samples! This is a common, and understandable, misconception. Population size alone cannot tell you how big a sample you'll need to consider (e.g. when planning a survey). It's also important to ask: "how variable is the population?". Suppose I want to know the most common car colour. If everyone drives the same colour car then, no matter how many cars there are, I'll only need to ask 1 driver. Of course you won't typically know the population variance (a measure of variety - the dispersal around the mean) in advance of your fieldwork. A practical approach is to look for secondary data which you can use to estimate the population variance. For modestly-sized research projects, a useful rule of thumb is that diminishing returns tend to set-in as the sample size increases above 30. You ought to have at least 30 results for each sub-group or segment you wish to analyse or contrast (bear in mind that once you've cut out the "don't know"'s you'll probably need more than 30 respondents to achieve 30 responses). Obviously for larger investigation - especially in novel topic areas where the secondary evidence is missing - there is rationale for larger sample sizes.
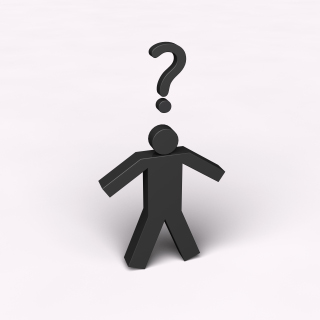
For further information I recommend BBC Radio 4's More or Less programme on which Tim Harford (the Undercover Economist) provides a sanity-check to the barrage of statistics quoted by the media: "More or Less is devoted to the powerful, sometimes beautiful, often abused but ever ubiquitous world of numbers".